Technology
The technology we have
Near Infrared Light + Hyperspectral Imaging + AI
Making the invisible visible
There is a region between 1000-1400 nm that is well transparent to living organisms
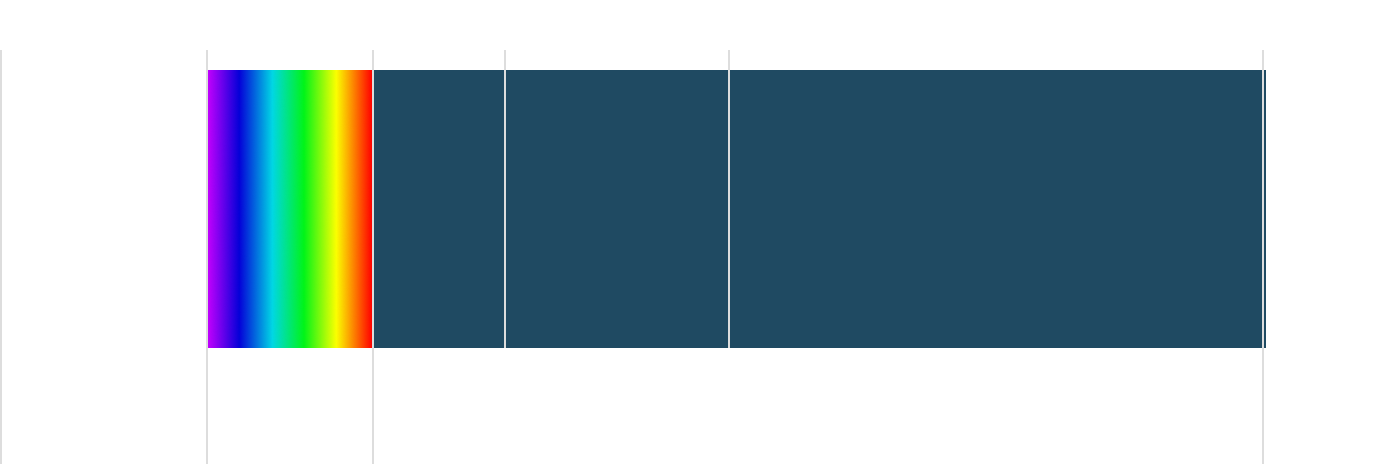
The light that humans normally see is visible light (VIS) in the middle of the spectrum.
The wavelengths between 1000 nm and 1400 nm are known as the "window of transmittance" for living organisms.
Our equipment can measure hyperspectral imaging (spectral imaging) from the visible light range to the near-infrared light range.
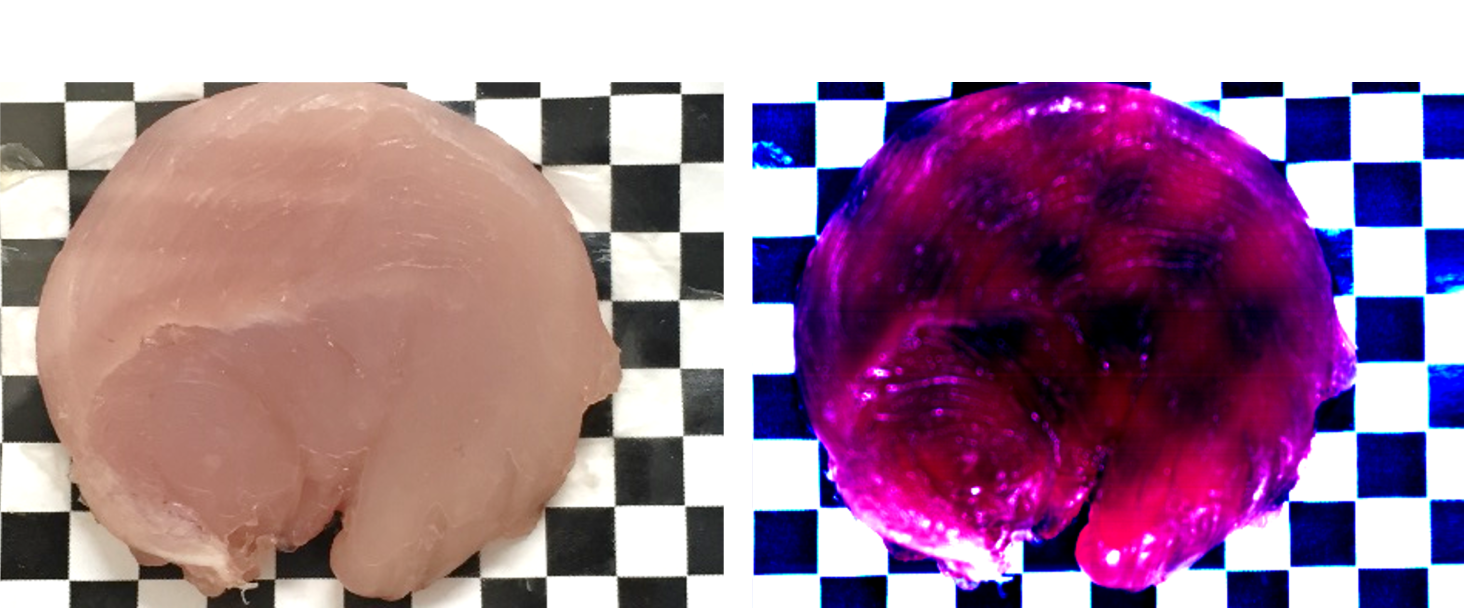
This is the whispering meat seen in near-infrared light.
You can see the checkerboard through the center of the whispering meat.
This is because near-infrared light passes through the meat and hits the checkerboard below,
and the reflected light is measured.
In other words, near-infrared light of a certain wavelength has the characteristic of passing through objects where light visible to the naked eye is reflected or absorbed.
(*Note: Near-infrared light is invisible to the human eye, so the colors are pseudo-colored.)
Hyperspectral Imaging (HSI) Spectral Imaging
A method of acquiring three-dimensional data with continuous spectral information at each pixel of the image
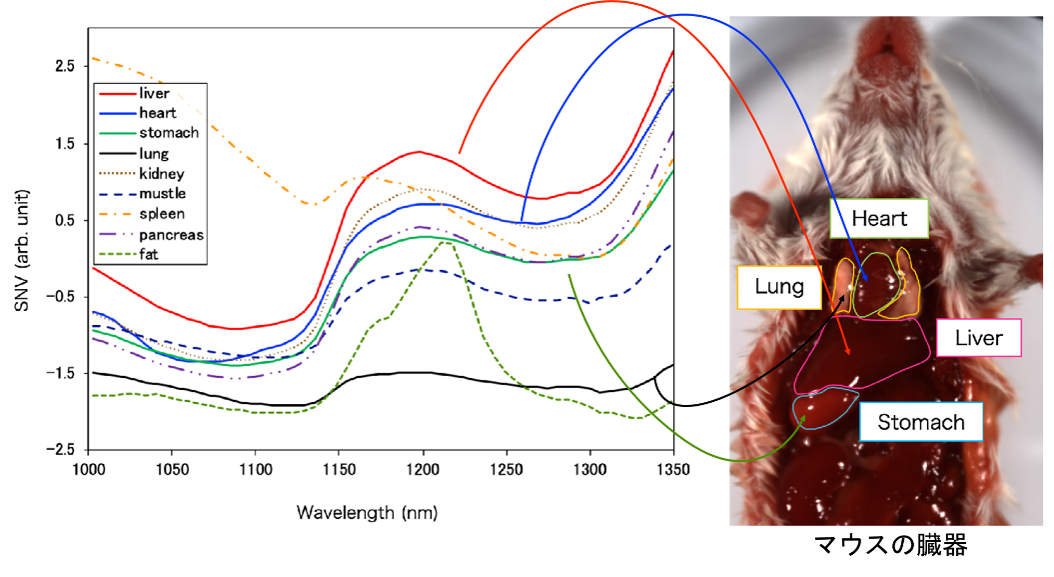
This is an example of an image of a rat's stomach. Each region (e.g., heart, lungs, liver, stomach) has a different absorption wavelength spectrum.
The color of the line represents the average absorption wavelength spectrum of each region.
Each site (e.g., heart, lungs, liver, stomach) has multiple pixels.
Each pixel has its own spectral data. (This is hyperspectral imaging/spectral imaging.)
For example, in a normal color image, one pixel (pixel) has three RGB values, but in this case there are over 100 values.
The graph shows that each spectrum is different.
Automatic Discrimination by AI
NIR-HSI can perform bio-transmission + component analysis + mapping.
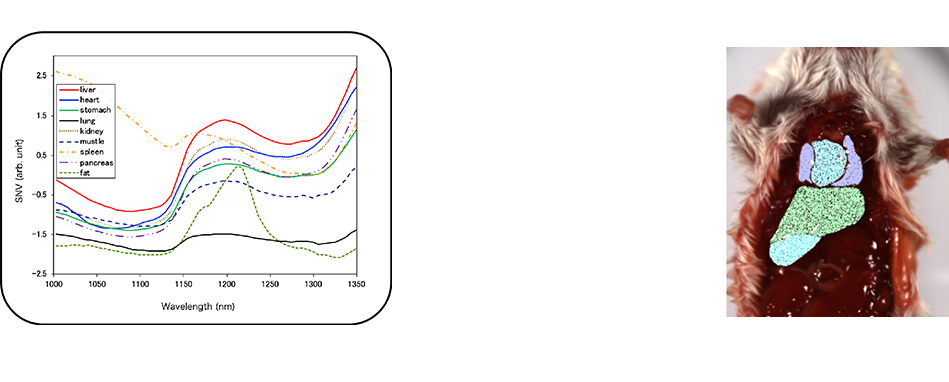
Since each pixel (pixels) has its own characteristic wavelength spectrum,
AI technology (machine learning) can be used to identify them.
Since data can be obtained for each pixel (pixel), it is a good match for AI technology.
The result of AI identification is superimposed on the original image.
The differences in color are the respective parts of the image. Since each pixel can be color-coded,
you can tell that it has been identified.
Measurement example: Visualization of deep blood vessels
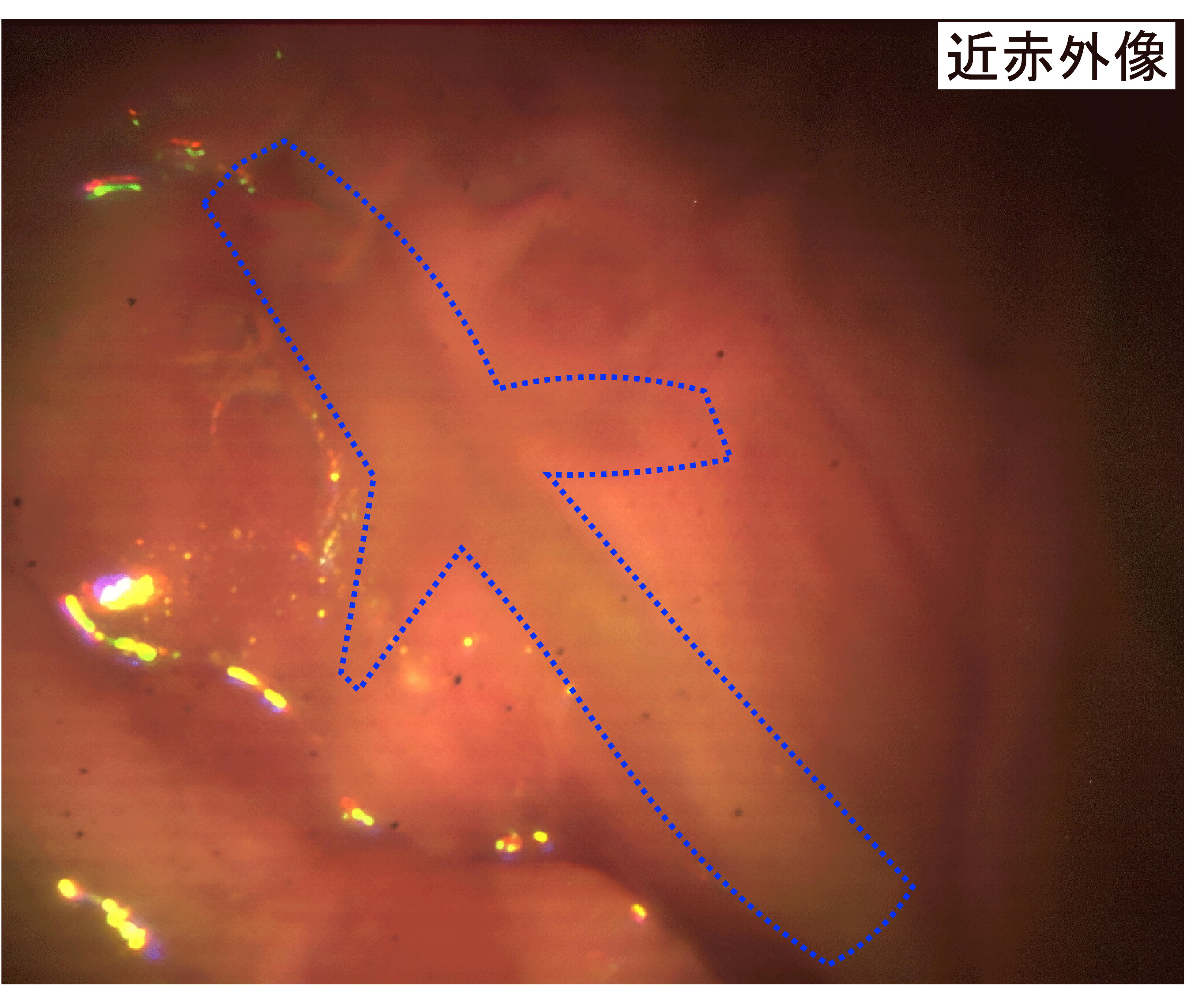
This photograph shows that endoscopic images taken during surgery can visualize large blood vessels from the superficial to the deeper layers of the body.
With human visible light (normal camera), only the thin blood vessels in the superficial layers are visible,
The thicker blood vessels (blue dotted lines) in the deeper layers are not visible.
In the near-infrared light image, the thin blood vessels in the superficial layer are not visible, but the thick blood vessels in the deeper layers appear to be raised.
Measurement example: Identification of plastics
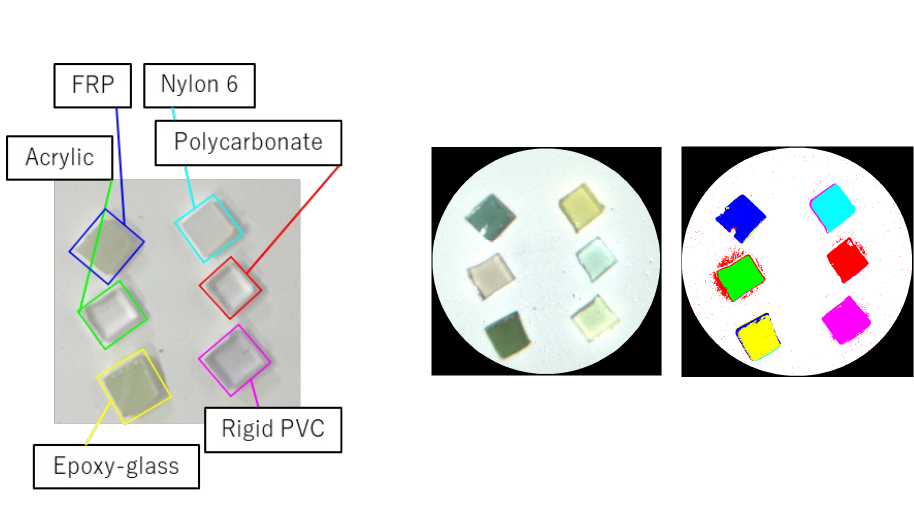
Examples of analysis of plastics measured with the NIR-HSI system for rigid endoscopes.
Left: The visible image shows six types of plastics that appear transparent.
Each plastic has a different wavelength spectrum to be identified.
Top photo on the right: Near-infrared images of plastics taken by the system. (The color is pseudo-transparent.)
Bottom right: Machine-learning-based identification of plastics captured by the device.
The color represents the identified plastic.
It is capable of taking and analyzing spectral images using visible to near-infrared light.
We have also developed equipment that can capture images of spatial ranges from micro (1 mm) to macro (1000 mm).
We are also capable of taking images in thin and narrow areas.
We are available for contract measurement, please feel free to contact us if you are interested.
For more information
"Development of a visible and over 1000 nm hyperspectral imaging rigid-scope system using supercontinuum light and an acousto-optic tunable filter," T. Takamatsu, R. Fukushima, K. Sato, M. Umezawa, H. Yokota, K. Soga, A. Hernandez-Guedes, G. Callicó, and H. Takemura, (Optica Publishing Group, 2023). https://dx.doi.org/10.1364/opticaopen.24882405.v1(プレプリント)
Abstract: This is the world's first report (preprint in press and currently under review) on the acquisition of HSI in the visible-near-infrared (1600 nm) wavelengths under a rigid endoscope. It was shown that spectra derived from organic molecules can be extracted by imaging and analyzing homochromatic resins and classified by machine learning.
"Evaluating the identification of the extent of gastric cancer by over-1000 nm near-infrared hyperspectral imaging using surgical specimens," T. Mitsui, A. Mori, T. Takamatsu, T. Kadota, K. Sato, R. Fukushima, K. Okubo, M. Umezawa, H. Takemura, H. Yokota, T. Kuwata, T. Kinoshita, H. Ikematsu, T. Yano, S. Maeda and K. Soga, Journal of Biomedical Optics, 28, 8, 086001, 2023. 12 pages.https://doi.org/10.1117/1.JBO.28.8.086001
Abstract: This paper examines the visualization of lesions containing non-exposed gastric cancer, showing that a tumor thickness of 2 mm or greater can discriminate between exposed and non-exposed lesion areas.
"Wavelength Bands Reduction Method in Near-Infrared Hyperspectral Image based on Deep Neural Network for Tumor Lesion Classification," K. Akimoto, R. Ike, K. Maeda, N. Hosokawa, T. Takamatsu , K. Soga, H. Yokota, D. Sato, T. Kuwata, H. Ikematsu and H. Takemura, European Journal of Applied Sciences, 9(1), pp.273 - 281, 2021. https://doi.org/10.14738/aivp.91.9475
Abstract: This paper describes a method for limiting the wavelength of NIR-HSI to reduce the computational cost of machine learning for deep lesion identification. It is shown that, for the identification of gastrointestinal stromal tumors that exist under the normal mucosa, the number of effective wavelengths can be reduced from 196 to 4 by machine learning with almost no reduction in accuracy.
"Over 1000 nm Near-Infrared Multispectral Imaging System for Laparoscopic In Vivo Imaging," T. Takamatsu, Y. Kitagawa, K. Akimoto, R. Iwanami, Y. Endo, K. Takashima, K. Okubo, M. Umezawa, T. Kuwata, D. Sato, T. Kadota,T. Mitsui, H. Ikematsu, H. Yokota, K. Soga and H. Takemura, Sensors, 21, 8, 2649, 2021. https://doi.org/10.3390/s21082649
Abstract: This is the first paper in the world showing that spectral images can be obtained at wavelengths above 1000 nm. By replacing a halogen lamp with a bandpass filter, it was shown that near-infrared light at 14 wavelengths could be extracted and used to classify tumor and normal areas in the carcinoma-bearing mice with 87.8% accuracy.
"Distinction of surgically resected gastrointestinal stromal tumor by near-infrared hyperspectral imaging," D. Sato, T. Takamatsu, M. Umezawa, Y. Kitagawa, K. Maeda, N. Hosokawa, K. Okubo, M. Kamimura, T. Kadota, T. Akimoto, T. Kinoshita, T. Yano, T. Kuwata, H. Ikematsu, H. Takemura, H. Yokota and K. Soga, Scientific Reports, 10, 21852, 2020. https://doi.org/10.1038/s41598-020-79021-7
Abstract: This is the first paper in the world to demonstrate that deep lesions can be identified using NIR-HSI. By performing NIR-HSI on an excised specimen of a gastrointestinal stromal tumor that existed under the normal mucosa and by machine learning, it was shown that a tumor covered by the normal mucosa with a thickness of about 2 mm could be identified with an accuracy of more than 80%.